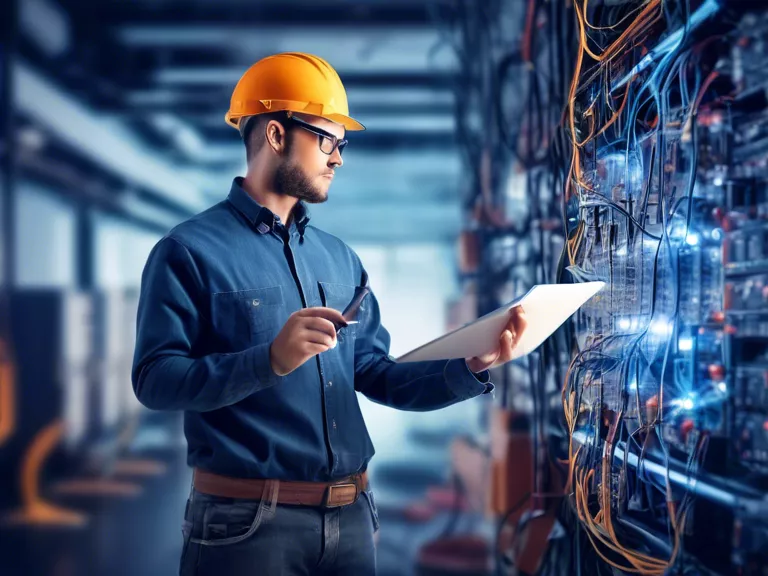
Machine learning is revolutionizing various industries, and the field of electrical services is no exception. Electricians can leverage machine learning algorithms to improve efficiency, predict maintenance needs, and enhance overall performance. In this guide, we will explore how electricians can implement machine learning in their field to streamline operations and deliver better services to clients.
Understanding Machine Learning
Machine learning is a subset of artificial intelligence that enables systems to learn and improve from experience without being explicitly programmed. By analyzing data and identifying patterns, machine learning algorithms can make predictions and decisions autonomously. In the context of electrical services, machine learning can be used to optimize energy consumption, predict equipment failures, and enhance safety measures.
Applications of Machine Learning in Electrical Services
Predictive Maintenance: Machine learning algorithms can analyze historical maintenance data to predict when electrical systems are likely to fail. By identifying potential issues in advance, electricians can schedule proactive maintenance activities, reduce downtime, and avoid costly repairs.
Energy Management: Machine learning can optimize energy consumption by analyzing usage patterns and suggesting ways to reduce energy waste. Electricians can use smart meters and sensors to collect real-time data, which can then be processed to identify inefficiencies and recommend energy-saving measures.
Fault Detection: Machine learning algorithms can detect anomalies in electrical systems by monitoring performance metrics in real-time. By flagging deviations from normal operation, electricians can quickly address potential issues before they escalate into major problems.
Safety Compliance: Machine learning can help electricians ensure compliance with safety regulations by analyzing data from inspections and audits. By identifying areas of non-compliance, electricians can take corrective actions to mitigate risks and maintain a safe work environment.
Implementing Machine Learning in Electrical Services
Data Collection: The first step in implementing machine learning is to collect relevant data from electrical systems, including voltage levels, current flows, temperature readings, and equipment specifications. This data serves as the input for training machine learning models.
Data Preprocessing: Before feeding data into machine learning algorithms, it is essential to preprocess and clean the data to remove noise and inconsistencies. This step involves normalization, feature engineering, and handling missing values to ensure the quality of input data.
Model Selection: Electricians need to choose the appropriate machine learning model based on the specific problem they are trying to solve. Common machine learning algorithms used in electrical services include regression, classification, clustering, and anomaly detection.
Model Training: Once the model is selected, it needs to be trained on the collected data to learn patterns and make predictions. Electricians should split the data into training and testing sets to evaluate the model's performance and fine-tune parameters for optimal results.
Deployment and Monitoring: After training the model, electricians can deploy it in real-world scenarios to start making predictions and recommendations. It is crucial to monitor the model's performance continuously and update it as new data becomes available to ensure accuracy and reliability.
Conclusion
By embracing machine learning, electricians can enhance their service offerings, improve operational efficiency, and deliver better outcomes for clients. From predictive maintenance to energy management, the applications of machine learning in electrical services are diverse and transformative. By following the steps outlined in this guide, electricians can successfully implement machine learning in the field and stay ahead of the curve in the rapidly evolving landscape of technology and innovation.
annemariealmedal.com
askdirtyjobs.com
aurora-f.com
best-selects.com
bio-sonix.com
blackchurchlistings.com
buzzofflucille.com
cashdoneeasy.com
centrictelecom.com
cyclo-clubdemours.com
defininghopes.com
discoveringawritersvoice.com
ecricketgames.com
evacoutodesign.com
hiasriasjkt.com
homework-help-secrets.com
jdpcreations.com
jnc-kk.com
jobs-top.com
kashmirhandcrafts.com
keunggulanwanita.com
marinegarageservices.com
outdooractioncompany.com
peaceinmedicine.org
perfumeultra.com
piphd.com
querosershark.com
sabatekle.com
shockworkgames.com
therapistschools.com
tiosbell.com
whatareyoucraving.com
willfaudree.com
agolfcart4u.com
andrealwells.com
bilgisayarmakaleleri.com
dadaduck.com
deadbeeteats.com
erikalinder.com
fluidcastvr.com
hollymccauley.com
icemanpolar.com
jerusalembiking.com
jsritterassociatesllc.com
kimonskin.com
lucihs.com
netatoridori.com
onlinebabydepot.com
pdp-tech.com
photoclubpdx.com
pietromarmo.com
poststarboy.com
property-sun.com
ps19school.com
rotize.com
scarlettattoos.com
shopjanayyatiece.com
speedxdating.com
spinesurgery-wecareindia.com
timsautoelectric.com
vseplotniki.com
wheelsandlenses.com
whenthegirlsrule.com
bohoandbowie.com
c-paths.com
captainscocoa.com
dziennikobserwatora.com
fallingfora.com
hf-advice.com
ilfarmacoacasa.com
kamigarciamargaretstohl.com
kdcleaner.com
kin-zushi.com
mangvietnamobile.com
mc-atwood.com
noelillart.com
teacherstechtoolbox.com
turknik.com
addisoncarmichael.com
attrition-game.com
bombaymotorworks.com
btbones.com
createandship.com
dbsnacks.com
dressporter.com
freshlovebirth.com
hikkirabo.com
jaipurjunctionhudson.com
kettlecoveenterprises.com
luckyfewbook.com
marrakech-hostel.com
motardspourlenfancenord.com
newfashionfantasy.com
phattoys.com
promomobilhondajakarta.com
reddapi.com
spanishvines.com
thehockeyworld.com
thierrycoulon.com
tracktownyouthleague.com
venom-ip.com
versaemergemusic.com
vyaparmantra.com
34thstreetsalon.com
anbointernational.com
appli4u.com
hubermovie.com
kazus2srq.com
lt-com.com
miss-south.com
mulholland-rp.com
phillyecocity.com
resumekerja.com
si-linux.com
sportspageteam.com
vvpllc.com
westernhealthhomecare.com
701themovie.com
alberguevegacarro.com
chameleonsthebook.com
crimsonaroma.com
custom-headsets.com
epiphyterecords.com
explorationmotherhood.com
gildawabbit.com
gorocketmarketing.com
hannawilsonphotography.com
heltio.com
hiveandbarrel.com
kangascafeandcatering.com
lilypadpos5.com
lonewaltz.com
militariamag.com
mobile-italia.com
oralefishtacos.com
paintsonladders.com
pickgenrealready.com
prepcorps.com
samanthaludlow.com
somelist.com
spooksandspirits.com
taskylonea.com
thebeauxmonde.com
thedroideffect.com
tlknighton.com
ultimateonlinebargains.com
urgentcare24.com
vintagechurchmvmt.com
vmarketerpro.com
bestscubapro.com
blackmoldremovalguide.com
blitztacklesms.com
cambridgearcsoc.com
centricperformance.com
crissyssalon.com
forwardkindheart.com
globalwomenqatar.com
hurleymetalroofs.com
igiveyoutheverbs.com
jfkweddings.com
komandda.com
kootenaygardening.com
laureenlivre.com
lifeservice-h.com
miraicampus.com
mystiquegrabber.com
neverradio.com
opalockahialeahfleamarket.com
ravenssouthend.com
restaurant-wars.com
skin-careclub.com
socraticproductions.com
stmaryinternalmedicine.com
studiobydark.com
teatteripaivola.com
travelcostaricaonline.com
vagrya.com
vivagoodlife.com
viviendasjardin.com
akfrontiertrappers.com
allreaderideas.com
auctionadcity.com
bioregistra.com
bridgersolarteam.com
club-hodgson.com
collegecounselorrowley.com
enfoquedemichoacan.com
hoja-hoja.com
hpplumbingsavannah.com
kristhescribbler.com
leftofstr8radio.com
lucyandsi.com
mattdebackere.com
metropluscolumbus.com
parroccazebbug.com
redditsuggestions.com
rembrandtquiballo.com
resonanz360.com
shareoslo.com
uppsalasciencepark.com
carsofsummer.com
ezytakaful.com
gamejamodb.com
herbertschroer.com
mograshop.com
aimieatkinson.com
arbreenarbre-mirabel.com
bajaringanjogja.com
benningtonplanningandpermits.com
blackbeartransmissioninc.com
boatcrunch.com
bobstiretown.com
ccidw.com
deafhoodmonologues.com
downtownsandiegochiro.com
generationcures.org
iotlinefair.com
jacksepticeyetour.com
jansenrestaurant.com
jobsciety.com
kcrestaurantltd.com
learnerblueprints.com
loscompadresgillette.com
maturewomanfitness.com
michaelskattum.com
mortechappliances.com
mothercluckerscomedy.com
rainer-turim.com
tampabaymusichistory.com
wallandroom.com
wannabananasnack.com
zeebioskop.com
avex-cjtv.com
base-displays.com
beyondfitnessclub.com
bodenboatplans.com
davemmett.com
dmtracker.com
emmaamigo.com
followmum.com
handblockprint.com
holstein-ojisan.com
honya-go.com
irontonwebcams.com
johnsjuicenyc.com
leangirlsclub.com
manifestdisc.com
natjecanjezborova.com
oxfordunimediasoc.com
protophotographer.com
pusatsewalaptop.com
recoverysock.com
reflexionscenter.com
tsukinihoeru.com
twintroopers.com
whitemilkgames.com
yashowme.com
alexmcfadyen.com
amahoro-music.com
arkansasgeographicalliance.com
blossomingthroughmotherhood.com
carlyrowenashop.com
chelsealecompte.com
desplainesautosales.com
etpatati.com
ezpettraining.com
generalroofsystems.com
glitterandgorgeous.com
greenecarclub.com
gsmheart.com
hodlvalley.com
intravenosa.com
iphoniac.com
karpanwhite.com
mdkzaman.com
myup4world.com
mywatcheshub.com
purewitness.com
richdadpoordadpdf.com
salisedine.com
supersports1017.com
thebudgetingtool.com
thepacktaggroup.com
townsvillecomputerrepairs.com
uncorkyork.com
unibioscreen.com
vyanama.com
dilijanschool.com
irent2u.com
mirainagasu.com
shakeittillwemakeit.com
suryasmara.com
3dgroundworks.com
ablelocksmithmiamifl.com
allaboutsunny.com
asrawi.com
caffedelreshop.com
chennaiclassify.com
contentcarry.com
corebusinessservices.com
courtneytight.com
fabianlawaz.com
grannysaudio.com
greygooseauto.com
hide-goldy.com
iblcardinals.com
karahige.com
kayfrydenborg.com
kimberley-kitchen.com
lakshmir.com
ldsfabric.com
manpowerrecruitments.com
marlinyachtsurveys.com
mindthemedic.com
mypowerdot.com
pahlaviha.com
picavoxel.com
planning-templates.com
promobonuscodes.com
rexchung.com
rustbelttoartistbelt.com
sb-cross.com
thatcherbuda.com
tjmartinell.com
acme-uae.com
alexcwilson.com
astraelevators.com
barefootandupsidedown.com
boladedragonz.com
brechogatopreto.com
dianamurdock.com
diijang.com
elitedamusculacao.com
falafullnyc.com
fm-view.com
francisquotes.com
gambillonjustice.com
glutenfreeinsd.com
ikokutabi.com
investidorvirtual.com
irevoltband.com
isolamaredmc.com
isoulshift.com
jasperfenceco.com
kegomall.com
kitchen-specialists.com
lafoole.com
m-ini.com
madeincocktails.com
marielacvetic.com
moteldesberges.com
nationalballoon.com
nerdtravelpro.com
nfbnlv.com
paperwolfdsgn.com
pupil24.com
simnasfikpunhas.com
snaccim.com
steven-nistico.com
syahidashukri.com
thebestaz.com
theyouniquepiece.com
toutetrien.com
vetobg.com
whitelight-media.com
xeireprint.com
babiestotoddlers.com
bajamarhotel.com
chucaokids.com
frejaswayceramics.com
gaihekitosou-hyouban.com
hansenpropertymanagement.com
hartleymiller.com
hayday-hack.com
keithcrossmusic.com
mikesalkind.com
munkvideo.com
musicosartistasycantantes.com
okinawa-emotion.com
oldlymeartsdistrict.com
panacearentals.com
pathshalanepal.com
patioumbrellaservice.com
sincerelykymberly.com
themenslab.com
ukbusinessprofiles.com
vaporizeremperor.com
visionarysouls.com
wasimthedream.com
wymeband.com
xpressloans911.com
alarbcoin.com
hajes-racing.com
mariamvenizelou.com
bookbeachbunny.com
viinahuuto.com
diet-bh.com
serhantmediagroup.com
secondhandpodcast.com
gravelhillbaptistchurch.com
washingtonprints.com
thelocalgoodness.com
olisticsmile.com
idealsweepstakes.com
artfulflight.com
uav-indonesia.com
claracavour.com
celticthundercruise.com
erinnorthcutt.com
teeruuk.com
mtlcuisine.com
aboriginalenvironments.com
islandjoescoffee.com
maglioncinoceruleo.com
motogadi.com
cyclistscientist.com
shea-karite.com
brownbettyteapot.com
perfectbusinessclimate.com
baycools.com
crazyotaku.com
art-imagery.com
boardandkiteafrica.com
dexautomation.com
ericdalen.com
feelbetterrehab.com
galabisnis.com
goshawork.com
hscleaningltd.com
kawdartgallery.com
littledanceinvitations.com
minottidesign.com
movieslider.com
natemccallum.com
network-experience.com
shoplelefashion.com
troquedeenergia.com
tyersandbeach.com
valentineall.com
visitadallas.com
yukiewithdownloads.com
21mill.com
aacdayton.com
astroatacama.com
avsmicroelettronica.com
bodybalancemw.com
cinemapremiere.com
cyclingdoubleheader.com
devseon.com
figurelovingfashion.com
jablonskimarketing.com
janecutler.com
jeremyfisherjunior.com
km2style.com
kosodate-diary.com
lawsonautoservice.com
locomprobene.com
mikhailmalyugin.com
redfortyeight.com
rogueflorist.com
ryanzone.com
sellyourhouseanycondition.com
sofiacybersec.com
theatlasinvestor.com
thedoubleclicklife.com
virgetemme.com
human-cell.com
konyoku.org
kr2forum.com
laudianelira.com
officialhollygibbons.com
onmyagenda.com
reprisalthegame.com
southbayinnandsuites.com
cinemapunjabi.com
criminaljusticereformjournal.com
ero-sta.com
harleysvillemedical.com
itneko.com
kandosaori.com
mix923neworleans.com
naradadalyan.com
solidworksgallardo.com
vintage-instant.com
allindiaads.com
businessbuildersbanquet.com
dallenbachanich.com
jmoceanavenue.com
reconciliationaccounting.com
theworthcampaign.com
boyin-bbs.com
boyinbbs.com
disprotec-ecuador.com
goodvillenews.com
inspirationdesigncenter.com
karlynsgallery.com
lionandrosesoap.com
nyschoolartgallery.com
safhaa.com
trentmcbride.com
afro-brush.com
arlingtonrefugeeservices.com
artonad.com
baykmadison.com
culture-talk.com
designerresaleconsignment.com
diptogo.com
drfrisch.com
dukeofdevonshirebalham.com
emission-online.com
freeoilcourse.com
hawaiifamilyadvocates.com
howtotiedye.net
iloveacu.com
kristahoefle.com
lillysbakeryshop.com
limbcmalaysia.com
loboads.com
mrpaulblog.com
myttoos.com
nickidenner.com
powerrabbi.com
rachaelmeddowsapps.com
robertodipatrizi.com
spaulforrest.com
tchad24.com
wefreelancing.com
whatispopularinjapanrightnow.com
yururuspla.com
zitunabanquet.com
batikkotagede.com
daimatsusushibar.com
julieohlemacher.com
oudaloud.com
sanasell.com
alliancejj.com
bahcekapilitekstil.com
corklab-ondo.com
customercarebest.com
giftsmacedonia.com
inscribe3d.com
jrnats.com
makemoneymakecents.com
mckinleyandpaget.com
nilzacosta.com
noithatvnn.com
pipedreamhempworks.com
sortedbook.com
warewelchwebhosting.com
wdccre.com
ymarryme.com
adinofcalifornia.com
afacerionlinereale.com
cheapohippo.com
clearbridgeaccelerator.com
deckdrainbesi.com
elfrashah.com
englishwifeindianlife.com
gueril-la.com
harvarddems.com
heatingcoolingcolumbus.com
jk-deai.com
kidsizesolution.com
leenchasedufour.com
luigismailorder.com
mahasupranatural.com
mozmassokomusic.com
scraptherapie.com
strawberrynaichigo.com
thenixons-afamilyportrait.com
thequaterz.com
uforeader.com
yamaguchi-satonomise.com
animalerielafinegriffe.com
diknows.com
doisongxeviet.com
duniainspirasi.com
f-enclair.com
fastprintco.com
forjaformacion.com
futureguests.com
indianremyhairextension.com
needlessthingspodcast.com
tatumsbagsoffun.com
tetu-tetu.com
woodlandsbedandbreakfast.com
crimestoppersrichmondvirginia.com
enprotec-inc.com
thebelairtreehouse.com
vegaatlangton.com
acepaintersdecor.com
bonzicity.com
budgettravelersinn.com
e-tosouten.com
personalizeittoledo.com
whisperingpinesosseo.com
wme-ae.com
185films.com
blazonsearch.com
broadviewmortgageorange.com
chrisadnittevents.com
dadnamics.com
effortlessseo.com
furlonglawgroup.com
greatcdo.com
hobodrifter.com
ichd-uk.com
indruc.com
jacklynlune.com
krissylynnvip.com
laura-simone.com
mindgymphilippines.com
orahono.com
petesbasement.com
phonefoldable.com
ratetheref.net
reviews-tutorials.com
skinnyapps.com
top10costarica.com
veldeypowersports.com
5misr.com
affordabledaycabs.com
aicbest.com
bolasabun.com
daindex.com
dogpatchpix.com
ian-pix.com
jimatpemikat.com
kbctvusa.com
neowood-editions.com
pattayagolfdays.com
pure-gel.com
qkslvrwolf.com
qzyik.com
seoragnarok.com
soccerproo.com
tempertoolkit.com
venturajeanseria.com
volaretranslations.com
webuyhousesfastsanjose.com
whipups.com
wilfridbastien.com
antimovski-han.org
anwardjaelani.com
aroundthewayapp.com
arsenalverlag.com
boulderhilltree.com
brightworkconsulting.net
cubakat.com
dazzlingwallpaper.com
diplomatsuites.com
drawboardvr.com
drjakefelice.com
eweekkorea.com
familylorefocus.com
firesafetysystemsva.com
huntersitesolutionng.com
hyo-go.com
jasonforsouthdakota.com
jedandlucia.com
lovedbylyla.com
mariemorenomyers.com
motorcyclestuffcheap.com
muskyhuntercatalog.com
pacihoken.com
polishatx.com
poltavhimmash.com
rabbirocketpower.com
share-de-common.com
storage-box.org
submityourbestarticle.com
szyniszewski.com
thejuiceradioshow.com
virginiabaseballacademy.com
webhostingfreereviews.com
yywestie.com
zillaspitbbq.com
albatrospelletteria.com
camechs.com
selflovetribute.com
wigglelondon.com
zackpetroc.com
authorspal.com
funkondatable.com
futbolperdidoenelmundo.com
greentanet.com
humblerootsorganic.com
kitnkate.com
lanshifashion.com
manbrainpodcast.com
markschwabinsurance.com
modernleaderscollective.com
renatodaiello.net
webgod.net
wouter-mijland.com
shojien.com
sane-way.com
3dlabtek.com
alexredondojoyas.com
allfairuse.com
bokutsuma.com
bondlakeac.com
canal150gramenet.com
ediscoverybuyersguide.com
euroautotrends.com
harleesangels.com
heavenly-chocolates.com
icitizenmag.com
jewelplayavista.com
lifesoperatingmanual.com
mommallennial.com
reviewta.com
sharingpctutorial.com
sheplanet.com
techringe.com
3cimaging.com
adventuresinhomeschooling.com
blatheringsblog.com
geekerymagazine.com
iso-sport.com
kokubunzidelyz.com
sotoblog.com
space242.com
tatikawapricolle.com
teraikoi.com
urbandesignreclaimed.com
wcsurgeons.com
arabesquespress.org
bigvilik.com
florida4marriage.org
risinghype.com
sito-web.net
35set.com
1077musicforlife.com
angelahubbs.com
arabic-html.com
bluestar-cafe.com
bon-aero.com
brunellagori.com
buylifeexperiencedegree.com
elchapulincoloradoanimado.com
elkmba.com
excelsupportservices.com
gilgameshproductions.com
kroniclesofkandk.com
mountainstatewrestling.com
murvual.com
myiconichome.com
noticiasdepenha.com
raisingthecameronclan.com
ronankhalil.com
rusverdict.com
saigon2cvtour.com
thesuperstarsband.com
childfinance.org
dreambigprintbig.com
eduresultsbd.com
marktwainonline.com
reversephonelookupfree.net
aokisports.com
cyber-idlers.com
filmchecks.com
hogrelspace.com
pope-michael.com
smile-camera.com
solacexis.com
teachingwhereyourecalled.com
thaoduochoangnam.com
timesalenow.com
anaffairwithitaly.com
dollfacelondon.com
originsofjudaism.com
raw-hollywood.com
rawopium.com
torc-anchors.com
automobileuploads.com
beritual.com
felinefm.com
freedateclub.com
gtmosquito.com
gullymovie.com
sequreinternational.com
stillwaterlogjam.com
watersnet.org
whatsnextsummit.com
zarehboston.com
bleachvsnaruto.com
eleventh-avenue-south.com
enterastream.com
foreverfreebyanymeans.com
futuregovnetwork.com
gerardooctaviosolisgomez.com
integritydefenders.com
leonardomanco.com
libreriasolaris.com
majalah-holiday.com
marsdiary.org
midmrkt.com
nobodyisperfectnyc.com
operationamped.com
pokechecklist.com
raspo-concept.com
semarangbagus.com
sikorskisausages.com
spitfirebars.com
successfuelformanagers.com
tjshomestylerestaurant.com
yayyoipo.com
cultural-materialism.org
kaitlinyoung.com
henry-danger-danschneider.com
avramrusuinteriors.com
cafeoggi.com
crosscountertraining.com
enriquecardenes.com
jjboe.com
mirianbar.com
momentumfitnessconcord.com
moonlightjourney.com
oregonstartups.com
silencethefoe.com
transcurebiosciences.com
travellingwithtania.com
alphacapitalny.com
babygiftshoppers.com
bigislandflow.com
bokionet.com
bouldermovementcollective.com
calatas-shampoo.com
calvertcentral.com
directglass-london.com
expandbizpromotionalproducts.com
fujimura-masanori.com
greatluxuryestate.com
jaltarang.com
lissersoft.com
messengerbotsforentrepreneurs.com
modivoice.com
montanarobes.com
mrscjacksonsclass.com
nigelburke.com
agrimedindustries.com
bromfordindustries.com
donotgogentlefestival.com
famille-soleil.com
focusvows.com
globsport24.com
gopushgo.com
hansinkan.com
jonnyarmstrong.com
joyparajoy.com
lavaughanjenkins.com
madonnadownload.com
maiko-movie.com
patchworkplanting.com
roemertopfllc.com
snakebytez.com
start-within.com
superfabpdx.com
televindu.com
thehushpost.com
thetoycabin.com
topqualityessays-aus.com
tritipman.com
undoshiftdelete.com
yikestoys.com
alqaem.org
backup-cable.com
bitcoinschmitcoin.com
charlestonarchive.org
cobaiaamiga.com
colouringbook.org
dancein.org
erniesautos.com
guardiansintent.com
jacksoncuppa.com
marefondum.com
mywimbo.com
nwafez.com
pbibcalella.com
penileguider.com
1159beach.com
casselsboykinpark.com
chamberband.com
divorcehelplegal.com
garagesaleacademy.com
gavanb.com
giltfounders.com
himejob.com
jaygoodys.com
kimberlymoffit.com
latinspirations.com
midmodcafe.com
mrnmnm.com
northadamsopenstudios.com
peachandplenty.com
pofcustomerservicephonenumbers.com
profinderroofing.com
searchinmena.com
shopwiznet.com
sunshinehempfl.com
swissclassicwatches.com
tanta2day.com
terkabul.com
thehollywoodmag.com
youth-live.com
annevictoriaphotography.com
awkwardlyaliveandpleasantlypeculiar.com
canvazify.com
columbiaboatalarms.com
dontplayplay.com
dreamworldafricana.com
faabis.com
g-roo7y.com
hannahpowellsmith.com
haryantokandani.com
hrdigitaltrends.com
iconpopquiz.com
irwingootnick.com
iuliabarton.com
karnatakaspider.com
kongwiz.com
maricjcs.com
mbkediadentalcollege.com
mizuki-shikimachi.com
nanukexperience.com
newstricky.com
otrtrailer.com
peak-dental.com
pearlthinks.com
ragazzeornamentali.com
seriesblueadventures.com
souvenirulangtahunku.com
theatreworksproductions.com
theworldroamer.com
tipspal.com
wallpapers3.com
24x7bookmarking.com
azureblogger.com
bungunote.com
creategoodmornings.com
cyclingdrenthe.com
entornomedia.com
eventsdoha.com
iklanbillboard.com
jennstrathman.com
tnotriskelion.com
alina-fedorenko.com
ballardcamera.com
bedstogosuperstore.com
cheese-experience.com
daniellekoste.com
hellomeganlee.com
hime-spa.com
isobe-stage.com
kellisaginak.com
pepstations.com
raysfiginfo.com
susanjablonmosaics.com
syn-biol.com
tarihin.com
terralunayoga.com
150psalms.com
activatearts.com
applce.com
appserver-zone.com
asp-zone.com
charactercrusade.com
dummaarodum.com
e30club.com
harborlightsrestaurant.com
lamothesurrogacyconsulting.com
mat-er.com
midwinterkitchen.com
puhlskitchen.com
rellacafa.com
saskatchewan-businessdirectory.com
sminno.com
storage9.com
therosenewdelhi.com
tinadoane.com
uuron.com
arabic-m.com
graviteklabs.com
immohunt.com
kangbedol.com
mediaexpertsearch.com
michellewesterlaken.com
owecraft.com
polettipiano.com
redstarsgear.com
s7nmusic.com
tinykingdomsil.com
viscoimages.com
Three Sided Football
All Student Deals
Buddy Whos
CBAHU
Dzeglebi
Gallery Wright
Harburn House
Jerry Summers Author
Knight Pulse
Melamina Fatima
Mesto Zlin
Metric Podcast
Nescio Seace
Paradigm Shift Therapeutics
Quantum E
Social Spacers
Ver La Ciudad
comedysearchengine.com
early-riser.com
flyingunicornstore.com
fujino-artvillage.com
fukuozzu.com
gofeverband.com
iamcendrillon.com
lareforma10.com
loonyletters.com
study-lyrics.com
trexentertainment.com
uncustomarybookreview.com
vinciblethedocumentary.com
airiworld.com
anthonyjdimartinojresq.com
bestrefrigeratorcentral.com
cardpaymentdirect.com
chinafalsecars.com
crudoprod.com
delightug.com
favor-j.com
fulltimefreedomweek.com
growgreenconference.com
gyakuten-pkth.com
heatherschnell.com
howyoupayit.com
ilpoteredellattore.com
izaksmells.com
kaataa.com
safecyclingaustralia.org
serialpulp.com
shelbynaturals.com
sleep-attack.com
stuartfergusonmusic.com
theokschool.com
tomgrimbert.com
tungbui.com
wolicreations.com
0-sam.com
app-zap.com
assiform.com
chocoforall.com
courtemanchecommunications.com
elkingtonfamily.com
energy-spirit.com
habbocreate.com
intelodoru.com
internetav.com
jerseyemblems.com
keylimecanada.com
montescolombia.com
ohsosweetbaker.com
rileyedgewood.com
runasnailspace.com
samsonos.com
yukariterakado.com
10okt.com
alexwardenphotography.com
bali-pintar.com
barbaragonzalezbarrera.com
cleopires.com
dewitt-operahouse.com
diquigiovanniandroni.com
exclusivelyyours-shopping.com
fukuoka-umanavi.com
jimhendersonpresents.com
lolipog.com
maplectseniorliving.com
omegastudiostest.com
pippaladoube.com
plantsenhance.com
prokachaimlm.com
raintreeterraceseniorliving.com
reed-jp.com
sakaeyasarou.com
tabiyukeba.com
thtbloodstock.com
toeicbook.com
wensskincare.com
yutakatoyota.com
zoedarrow.com
american-boffin.com
bfbchamp.com
democraticcoma.com
tigrepelvar.com
charpoles.com
derbywheelblazers.com
fansfocus.net
guildnow.com
hediyeteyze.com
isprimecdn.com
kiira-korpi.net
manutd24.com
mediumtylerhenry.com
mishanghai.org
savethreestrikes.com
smilesbydavis.com
10puntos.net
band-shirt.com
icelandtrails.com
paulmarioday.com
thefunnynanny.com
bradycallahan.com
cahillfilms.com
freshmilkmama.com
ieero.com
imacert.com
ioka-boxing.com
kennethlightstudios.com
mesoraware.com
nespa-jp.com
onenessjewelry.com
onlinepluz.com
patproject.com
sbwritersconference.com
villaggiosabbiedoro.com
yj-plusalpha.com
sansaranepal.com
adriantai.com
bruno-surdo.com
camelmilkmagic.com
chateaudelapommeraye.com
cjmadolara.com
crestcalicut.com
dianadworak.com
dosankonet.com
elsecretodelaluz.com
gaboleon.com
gotthetravelbugtoo.com
greenesrelease.com
holleyrubinsky.com
iamsinofficial.com
infinitytimewatch.com
katielangin.com
micro-mosaic.com
mygetrealtoheal.com
netz-suruga.com
photographybymerrilee.com
pike-plan.com
playaugustapines.com
rahcmusic.com
rimukobo.com
sardiez.com
sheepser.com
shipshewanabakery.com
studyaims.com
theecotoneexchange.com
thefitnessguild.com
wetalkhealthy.com
agrogrua.com
ainori-izakaya.com
aveirotuna.com
c-drew.com
callummillward.com
dimitridube.com
excelcondo.com
fltjets.com
flyinaway.com
fun-cycling.com
hadleighmarket.com
lazlobane.com
lookaboat.com
madievals.com
mulliganandbanham.com
namesdublin.com
naskobbystudios.com
ridenowcraig.com
southfloridastriders.com
subkuchpk.com
toyodamingei.com
urbanflorava.com
via-tecnologica.com
vonzella.com
whitenmysmilenow.com
beauty-galore.com
childrensneighbourhoodsscotland.com
content-gbl.com
cosmocurioso.com
deliheru-station.com
euromabcr.com
hagiplan.com
handmadebyanadel.com
hcnaturalcare.com
iwucom.com
jdirect-kaiyoso.com
kishikarate.com
miere-manuka.com
missnoise.com
mjwjtalkradio.com
nazilaloveglamour.com
notananimal.com
onnanougyoudo.com
pendleboard.com
politicsunspun.com
rvmekongnavigator.com
scarydairypress.com
scrapattackscrapbooking.com
splitube.com
thebargainbins.com
thedukesmenofyale.com
thekoolgadgets.com
aksato.com
computerliquidationstore.com
createnew-account.com
evgeniybulatnikov.com
logandumpbodies.com
marcoradeschi.com
mileagejunky.com
ohiowins.com
progauntlet.com
thegemladyinlondon.com
venezuelandays.com
al-marzook.com
alphatechsim.com
alumgalvez.com
fanisnews.com
kabeljoes.com
shopitla.com
water-tsarbet.com
worldbaseballhierarchy.com
yourpleasurepoint.com
zvikico.com
bebovino.com
car-trackers.com
colbykeller.com
companyregistrationuk.com
dayoftears.com
entamee.com
kuttikattil.com
litjunkies.com
ljshen.com
nostalgic-radio.com
pcgameshackcenter.com
rachelboggia.com
vanessagritton.com
veraandrose.com
vernissage-zermatt.com
Breadboard Maniac
Takasu App
Anostomodo
Bonet Scissors
Cora Viral
Dippity Do Dog Mobile
Group Tie
Newberry County History
Oslo Velo
Planned and Present
Wilshire Language
CFL Magazine
Corner Kitchen
Glass of Win
Hydeout at the Wharf
Lotus Relocation
My Dee Dee's
Platinum Avtomaty Wulkan
Pride Outside
Rollout Reviews
Smile to Africa Adventure
ABC Trcker
Aloha Botts and Tots
America Noticias
Are You Kenilworthy
Ask My Condo
Charm Shiki
El Akhbaar
Health Cursor
Home Again Creative
Mi Punto PE
Patt and Billy
Rustique Online
SS Pro HK
Steamworks Espresso
Targeted Fat Loss Training
Team Hamano
Used Cars Buying
Dave Tries Ballet
Buon Grande
Criacao Sites
Perry Perkins Books
Writing Essay in AU
Ka Soku
Blood is Blood Movie
Eleanor Writes Things
The Happy Prince Beirut
Town of Witless Bay
Online Igrovoi Club
Trigeminal Neuralgia - Ronald Brisman MD
Chocolate City Burlesque
Advanced Electric Scooters
W Tougei
Aljouf Now
Arrister
Bruce Holmes Construction
Burlington Bridal
Chocolate Creative Design
Culture of Life Store
Elizabeth Cryan Photography
Fun Fresh Ideas
Kotoyuujin
Lyudia
Nakakirei
Peer AGS
San Diego Vacation Service
Sinsei PV
Think Tank West
Wimax Gogo
Asperger Way
Book Rack WA
Hume Coover Studio
Pico Compressor
PPTAA
Artina Films
Bash Booths
Bicher Cancer Institute
Brickyard Theatre
Coffee with Jeff
Essential Libertarianism
Find the Light Within
Font Plus Tips
GB Product News
Homer's Auto Services
Luxellence Center
Matome Hannou
Nepal Smoking Pipe
OEM Korea Brand
Shoe Saxo
The Stock Market Blueprint
Untapped Inc.
Andres Carizza
Ashland Aerial
Brown About Town
REH Shows
Shotover Country
Toni Halonen
Trainers P
Gaya Hidup Sehat
Bulging Disc Fixed
Cadogan Cosmetics
D Chavannes
Kilo Pages
Loring Military Heritage Center
Modular Homes MA Planning Center
The Wheel Herb Farm
Bxtches Be Blogging
CCTV Installers London
HiFi Noise
iGo Inter
Payroll Services UK
Shop Moraga First
The Highline Restaurant
Visval Bags
Mantis Plant Protetion
Photography Travel
Desperate Endeavors Movie
Delight Worthy
Unryuuji
Wamsted On Energy
Jual Aged Domain
Gimena Macri
Vietnam Tours Lotus
Kingston Single Malt Society
Escape the Stall
Pro Point Dies
Basement Metal
Boeren Boedel
Lucky Dog Grooming and Boutique
Nihon Megane
Osiris The Series
Suzy Wimbourne Photography
The Hollywood Garage